Why we love experiments
We run so many experiments because they are a structured way to test ideas, and only put more effort into a business if we have built a strong body of evidence to support it.
In this series, I’ve been walking you through the theory underpinning rapid experiments in the world of startups, and examples of cheap, fast experiments you can run as soon as today.
But now it’s time to put these lessons into practice, and show you how we run successful experiments at The Delta using the test card.
A disclaimer: a successful experiment isn’t one where you were right, it’s one where you ran the experiment the right way. This will become clearer later on.
Before we jump in, if you aren’t entirely sure why experiments will change your life as a founder or a member of a startup, are unsure of the theory underpinning the lean startup movement, and don’t know any of the common experiments that startups can run, I recommend you read my last three guides:
Let’s now cast our gaze towards some of the experiments we’ve run alongside founders at The Delta, and the learnings the venture team took from them.
Validation Survey Experiment: Fine-dining delivery service
We envisioned a service that would allow people to have multiple-course, fine-dining meals delivered to their doorstep in time for dinner, allowing customers to enjoy a fine-dining experience from home during the pandemic, while supporting local fine-dining chefs.
The venture team wanted to, through a survey, see whether customers would be willing to pay enough for this service to be profitable, and also whether customers would make use of the service often enough.
Metrics to monitor were the average amount customers would pay, as well as the average frequency that they would use the service (e.g. twice a week, once a week, twice a month, and so on).
Here is the test card we designed for this experiment:
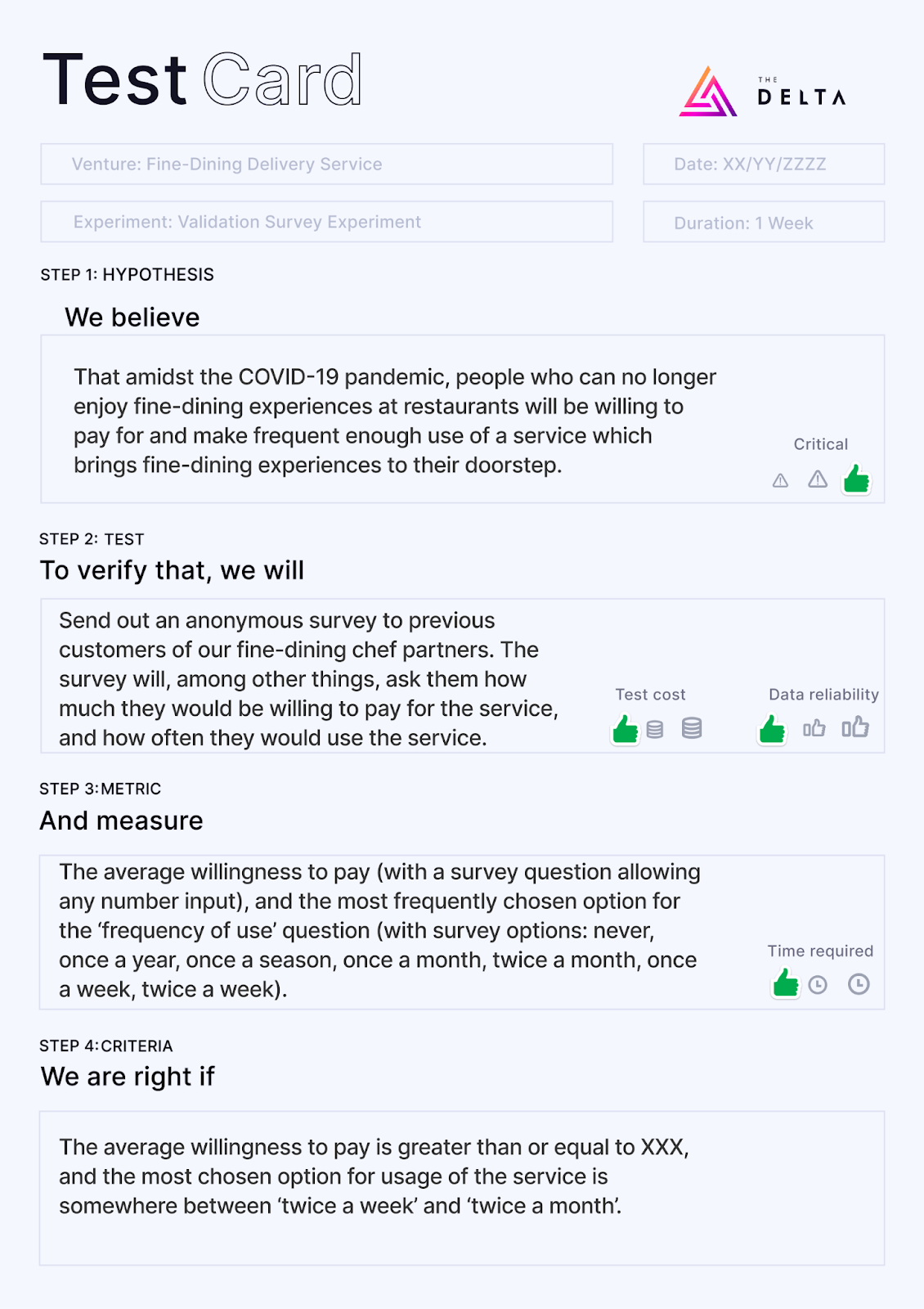
What did the results say?
- The average amount customers would be willing to pay was markedly lower than they would for a sit-in fine-dining experience.
- They would only use the service once a month.
What did this prove to us?
The unit economics of running this venture no longer made sense - overheads like technology, the platform fee the service would take from chefs, and delivery meant that the margins would be incredibly low; on already small volumes because it was a higher-income target audience who had just stated that they wouldn’t use the service often.
Overall, desirability and viability were low, even though we could technically build the product if we wanted to.
Good thing we found this out before building the platform!
(Unsure of what we meant in the test card by ‘test cost’ and ‘data reliability’, and how you could work these scores out? Read my guide on experimentation theory to get up to speed)
Landing Page Experiment: Local discounts application
We got quite excited by the thought of an application that would let people ‘recharge’ after a long week by giving them large discounts to a bunch of weekend-only, local experiences.
Before building the app or contacting dozens of brands to organise discounts, the venture team decided to drive traffic to a website to see if there was interest in the idea, determined via a waitlist.
The team targeted people with high-power, high-intensity careers, so LinkedIn ads were used that referred users to a simple landing page, which had a clear call to action to join the product’s waitlist, as well as static content further supporting the product’s value proposition.
Here is the test card we designed for this experiment:
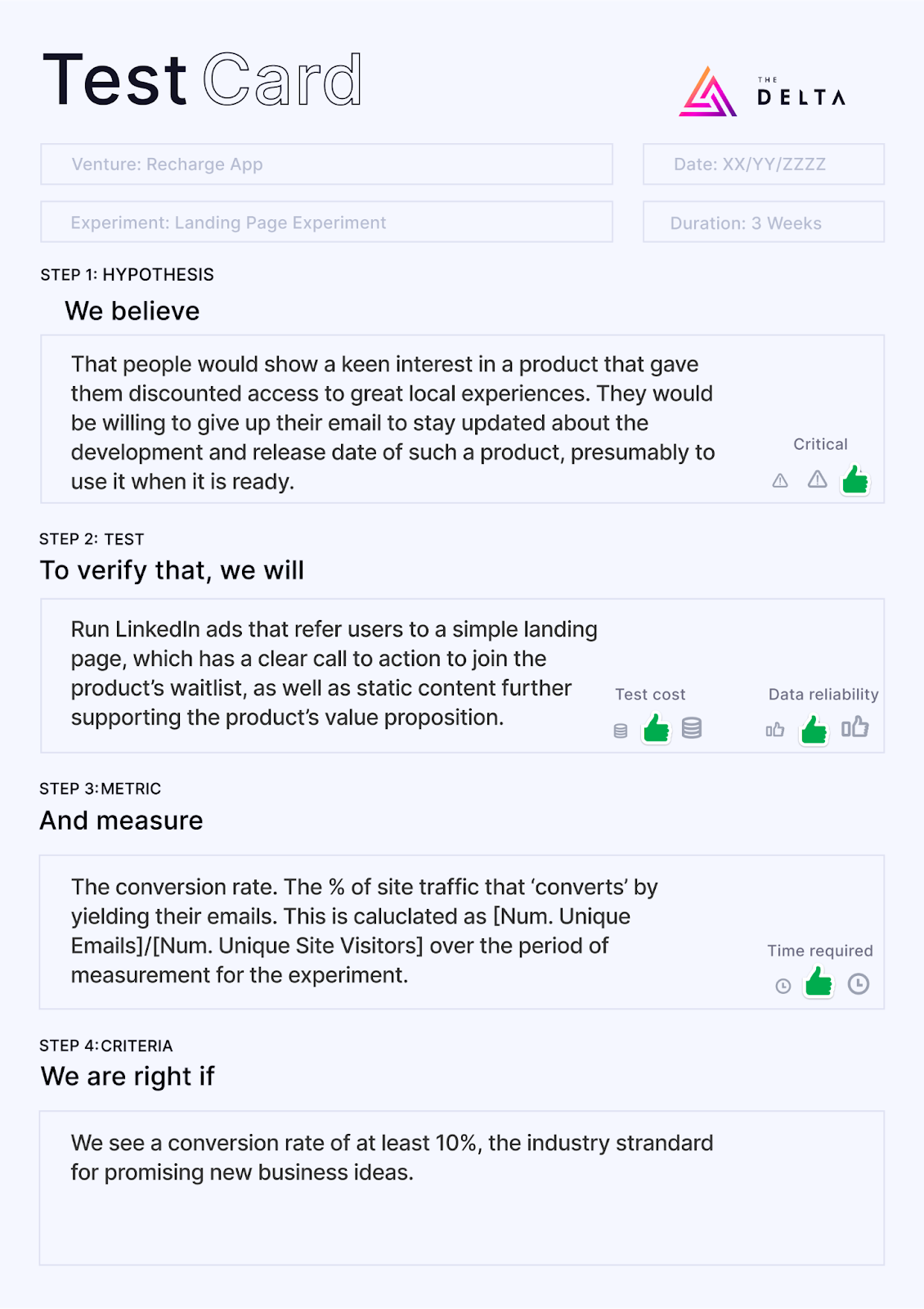
When the conversion rate on the landing page to join the waitlist ended up being between 20% and 25% (a typical standard is 10%), we were really pleased with the result and the several hundred early users we had secured. There was a good indication of desirability.
However, a separate learning from the experiment was that the cost per ad click (CPC) on LinkedIn was incredibly high. We would either have to explore advertising on Facebook (which is typically cheaper but has fewer targeting options for specific types of occupations), or face the reality that, though desirable, the venture wasn’t viable - people wanted it but we couldn’t build a sustainable business model out of it.
Wizard of Oz Experiment: Market research platform
We wanted to build a survey platform that allows businesses to set up a survey and then have it distributed, via WhatsApp, to an audience of low-income customers - to conduct market research on them.
This would have been strategic to our own business where we conduct lots of research, but also a product with wider use to the research industry.
The venture team could not afford to build a WhatsApp bot or automatically conduct data analysis on the results at that time. Despite that, we had hundreds of survey respondents on standby, good data science skills, and we just had to ascertain whether companies would pay for the market research assistance.
To verify this, we conducted research campaigns for five clients for free. We took their surveys and manually asked the questions to our respondents, via WhatsApp, until all the data was in. Then we created a dashboard of the results in Google Data Studio and sent it to the clients, creating the impression that all of this had been done by our system.
We had decided that if at least three of the clients (60%) were willing to pay for a second research campaign, the Wizard of Oz implementation would have been validated and we could start preparing to automate more of the system, such as building a WhatsApp bot.
Here is the test card we designed for this experiment:
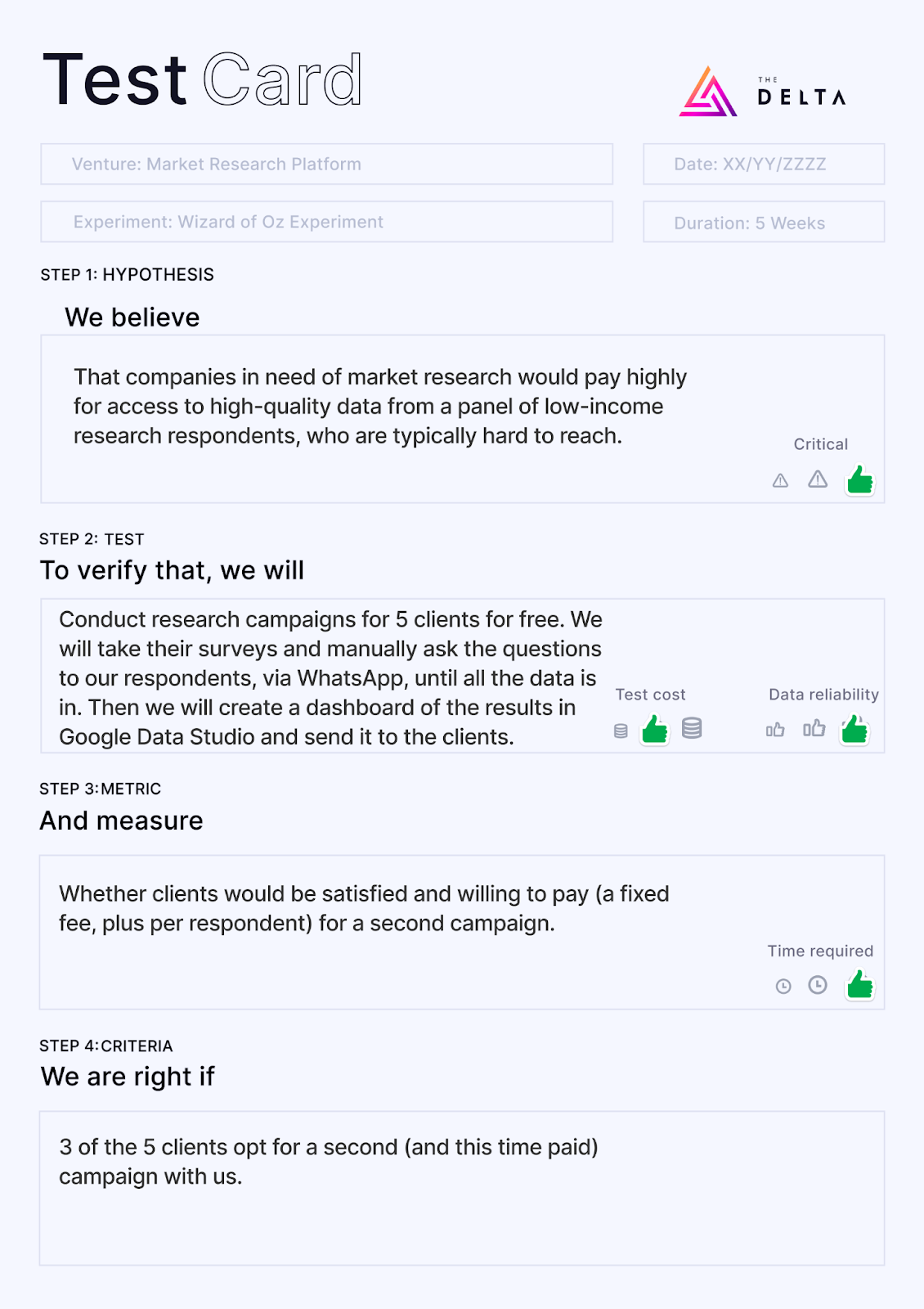
Fortunately, all of the clients were happy, and paid for follow-on experiments, and now this is a well-funded venture with dozens of clients, and on its way to changing the market research landscape!
A/B Test Experiment: Gamified donations platform
We worked on a gamified-donations platform that would reward people for donating to worthy causes, by entering them into prize draws. While the early charities to partner with were fixed, the venture team was unsure of which prizes the platform’s audience (low-to-middle income adults) would prefer.
The team ran Facebook ads that showed three different types of prizes (cash, technology, travel) of equivalent value, to see which prize attracted the most interest, as an indicator of which prize would have the best unit economics if a live prize draw was done.
Success was defined as one of the prize types drawing in 50% or more of the clicks, or being declared as an ‘early winner’ in Facebook’s A/B testing interface.
Here is the test card we designed for this experiment:
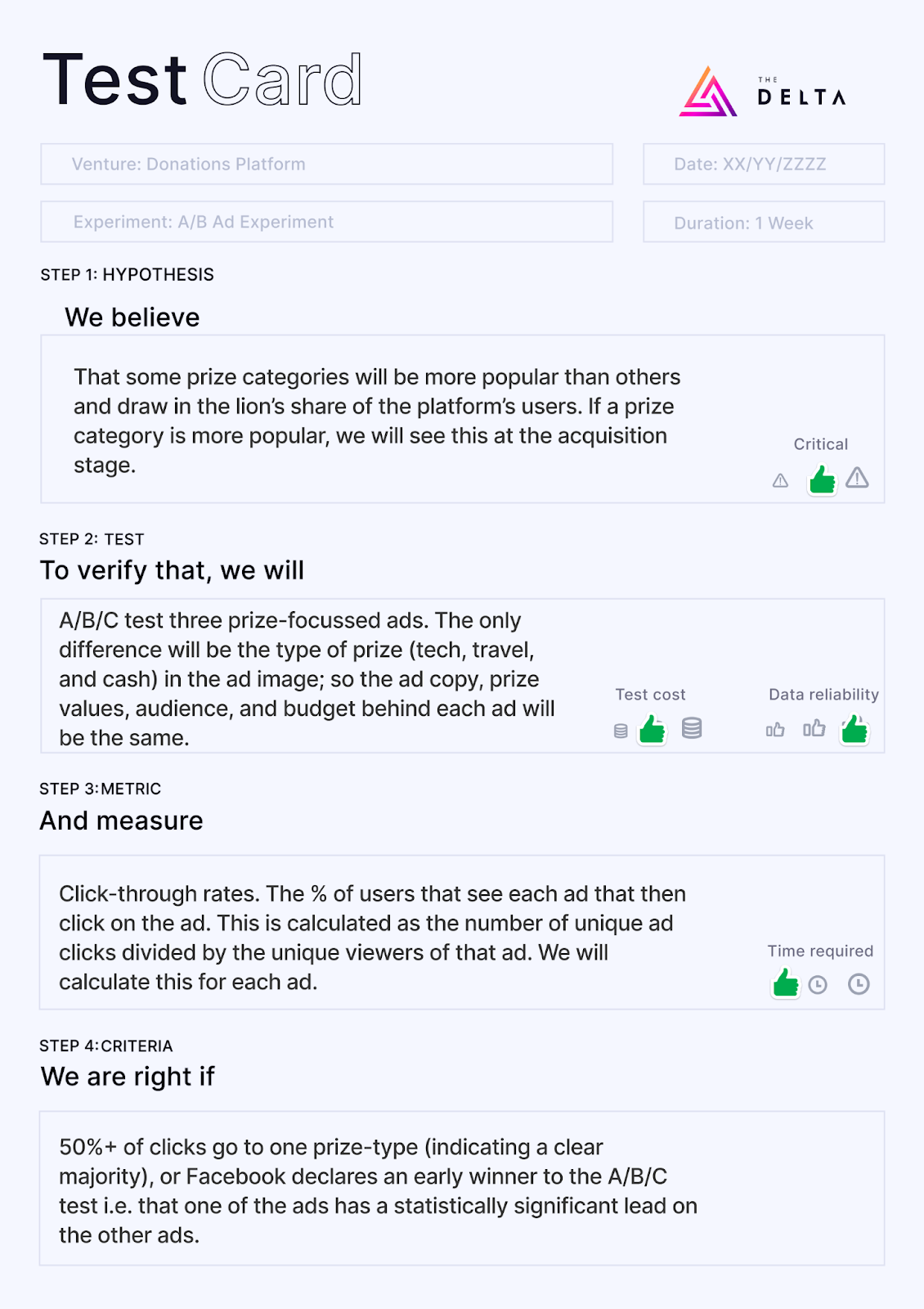
Ultimately, the technology prize was declared as an early winner and we went into our first prize draw with a validated prize on offer.
There is one mistake we made during this process, however.
We ran a second ad experiment where not only the prize types (cash, technology, travel, fashion, cars) differed, but the value of the prizes. By testing two variables at once, we couldn’t conclude on why certain ads performed better than others. If the fairly cheap PS5 performed better than the expensive car, was it because our audience fundamentally valued technology prizes more than cars, or because they thought they had a higher chance of winning a small prize?
Read my earlier guide on experimentation theory to see a list of common flaws in the design of experiments.
Concierge Experiment: Personalised nutrition product
We designed a personalised nutrition service that would allow young customers to take a short quiz, get a recommended blend of ingredients suited to their unique circumstances, and then order a custom supplement powder containing all those ingredients, to their doorstep; with the option to resupply in a month’s time.
The venture team understood nutrition well enough to make recommendations without an algorithm, so before building out a sophisticated algorithm or automated end-to-end eCommerce product, we wanted to get a sufficient number of satisfied, paying customers with unscalable, manual methods.
We made 30 manual sales to customers (about 3-5 a week), where we got them to do the quiz, gave them a recommendation within 24 hours (they knew a human was computing their recommendation), took payment, and then delivered the product to their homes within 5 working days. Afterwards, we asked them to do a satisfaction survey.
We used a metric called the net promoter score (NPS) for this experiment, where you ask a customer how likely they would be to recommend the product to a friend, on a scale of 0 to 10. People who give a rating of 0-6 are ‘detractors’, 7-8s are ‘passives’, and 9-10s are ‘promoters’. The NPS is calculated as the % of Detractors subtracted from the % of Promoters.
Here is the test card we designed for this experiment:
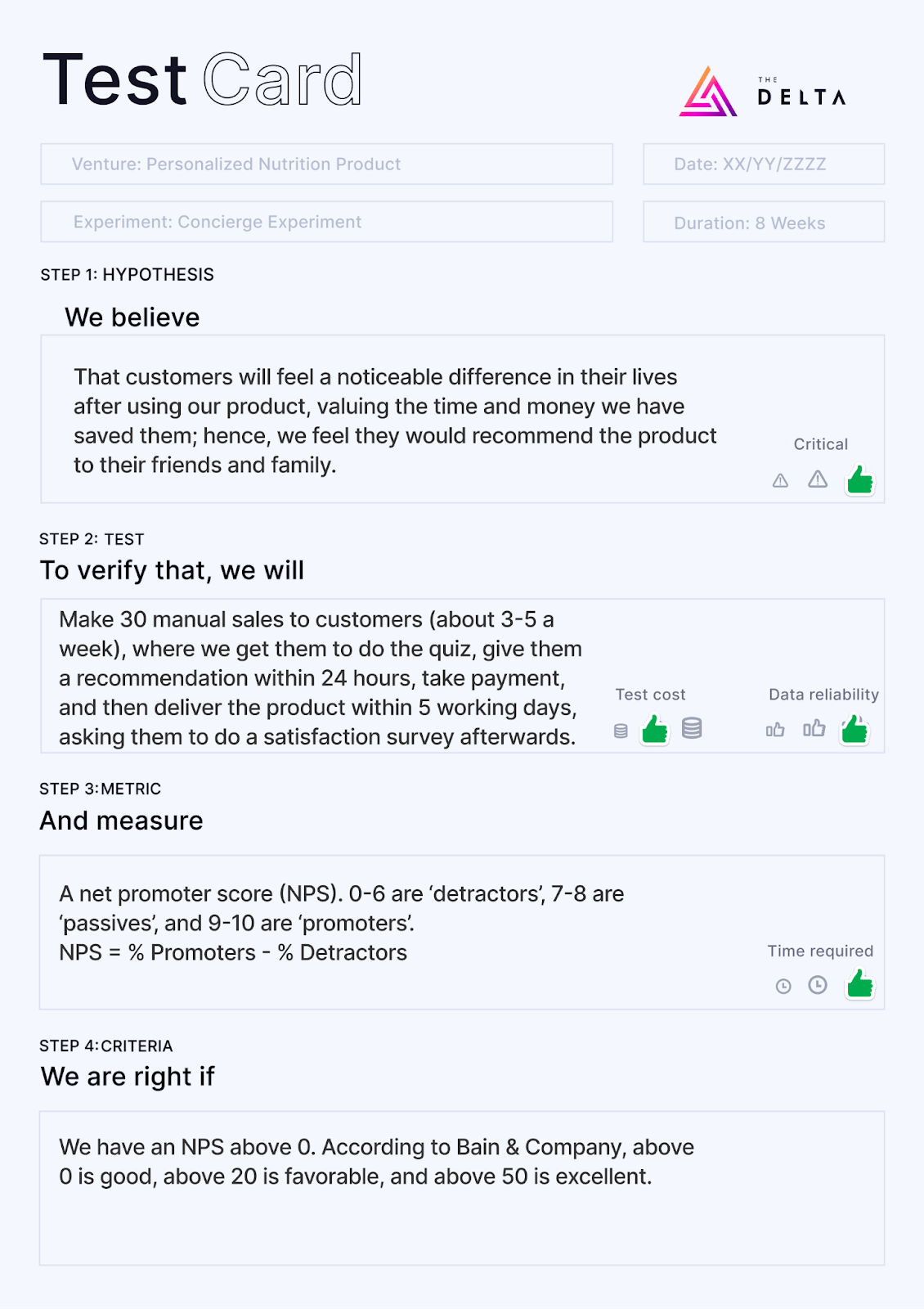
According to Bain & Company, above 0 is good, above 20 is favourable, and above 50 is excellent. After running our concierge experiment, we got a score close to 0 on the negative side.
This begged the question of whether our low customer satisfaction came from long waiting times on a recommendation and delivery (which would have been improved by automating our processes anyway), or was related to fundamental issues with the supplement product that was delivered.
When we discovered that there was dissatisfaction with the actual supplement, our ‘killer feature’, we knew the venture had no legs.
The ease of selling the product manually indicated good desirability, but if the product provided by our only available custom nutrition supplier wasn’t up to customer standards, we would not feasibly be able to service that desire.
And even if customers were ‘okay’ with the product, they would not become repeat customers nor would they be likely to refer it to friends, which spelled doom for its growth/viability prospects.
You can’t build a sustainable business out of only making first-time sales, especially without referrals. The CAC would be too high and the LTV too low.
The importance of reflection
What these case studies have hopefully illustrated is that hypotheses aren’t always correct, but if you’ve followed the right experimental approach, you have to accept the answer and use that as a point of reflection.
To keep the post-experiment analysis structured, each test card is eventually accompanied by a ‘learning card’, where we detail the outcomes/observations of the experiment, the learnings we can extract from that, and the next steps.
Now that we’ve introduced you to both our test cards and learning cards, feel free to use them as templates for your own experiments:
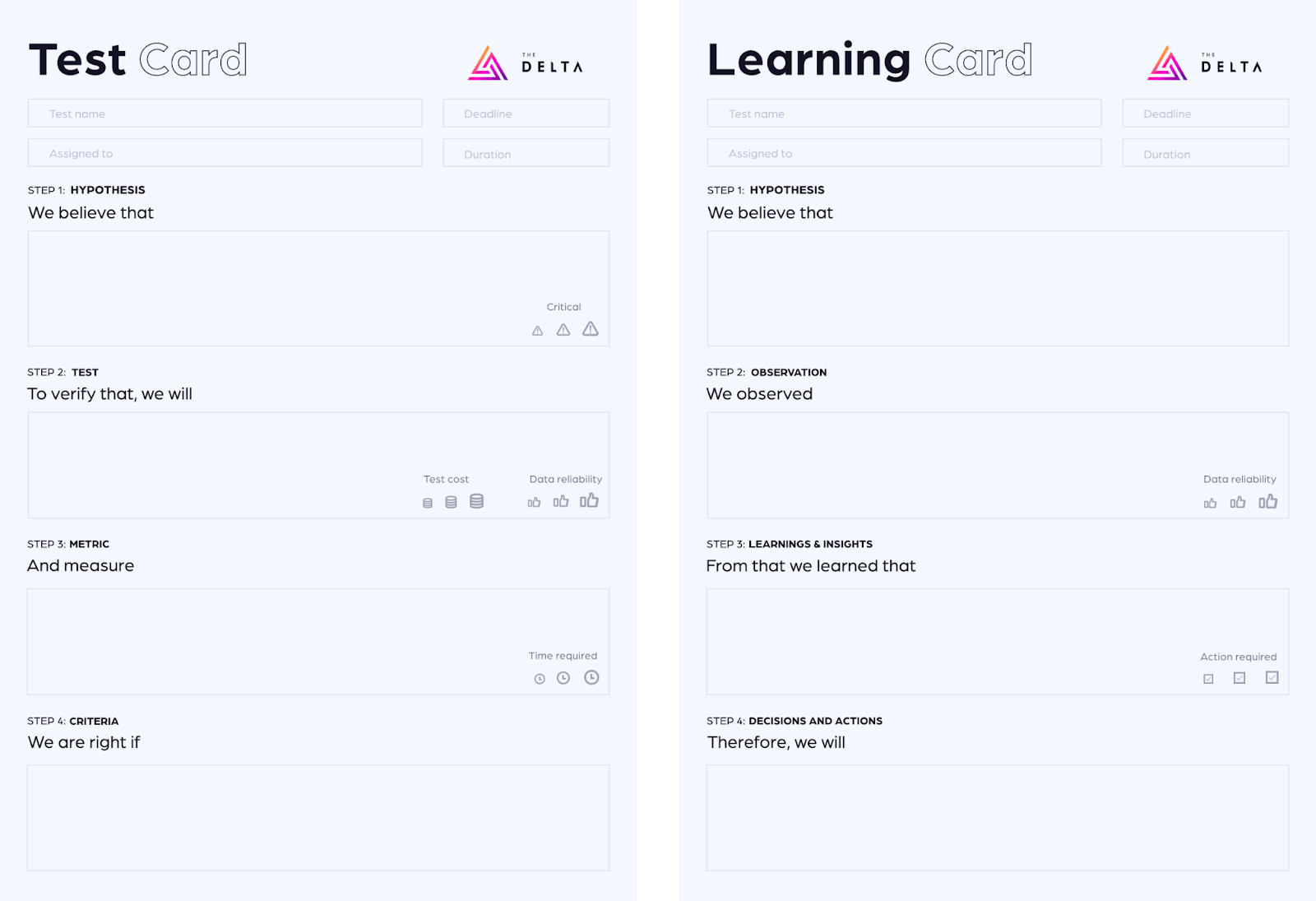
As a last note, in some cases you may feel like an experiment rules out your idea too early, and that you’d like to test your hypothesis with a different experiment - this is valid, there may be false negatives in some cases; but over time we’ve observed that it’s always good to start small.
Rather run a small-scale experiment with a risk of a false negative, than run a large-scale experiment only to invalidate assumptions that could have been tested cheaper/sooner.
Ready to get going with some experiments? If you haven’t already, check out my last guide for a list of some of our favourites.
Hopefully these resources help you become more confident and comfortable with running experiments.The next and final guide in this series will explore the emerging low- and no-code fields, and how they allow startups to run experiments and build MVPs way better than ever before!
At The Delta we’ve validated hundreds of ideas for early-stage entrepreneurs and unicorns alike. It’s what has helped us curate a venture portfolio worth more than €3.4bn.
If you’re a founder, startup, or scaleup with a great idea for your next business, feature, or product, and are unsure about how you can get running with it today, talk to us and get a free consultation with one of our top strategists.
Validated your idea already and ready to build something? Explore how we take ideas through design, development, and then eventually launch them to the market.